Meta Unveils Llama 4: A New Era of Open-Source AI Models
Meta Llama 4 delivers a highly powerful open-source AI with MoE architecture, featuring Scout, Maverick & Behemoth for top-notch scalable multimodal performance.
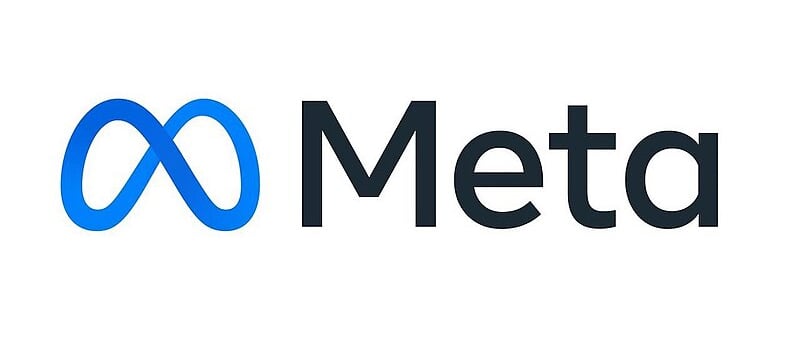
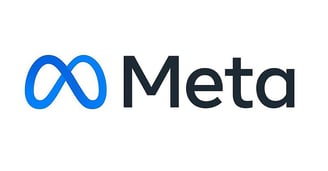
Meta has once again made waves in the AI community by releasing the latest generation of its Llama family on a Saturday—underscoring the company’s urgency to stay ahead in the competitive landscape. The new Llama 4 models mark a significant evolution in Meta’s open-source approach, introducing groundbreaking innovations in architecture and efficiency. In this post, we dive into the details of the three main models announced: Llama 4 Scout, Llama 4 Maverick, and Llama 4 Behemoth.
Key Highlights:
Efficiency & Accessibility:
Scout is designed to run on a single Nvidia H100 GPU using Int4 quantization, making it an accessible option for developers and researchers without large-scale GPU clusters.MoE Architecture:
It employs a mixture-of-experts (MoE) approach with 16 experts, activating only 17 billion active parameters from a total of 109 billion. This selective activation optimizes resource use while maintaining high performance.Unprecedented Context Window:
With a 10 million token context window, Scout can process vast amounts of text—ideal for tasks like document summarization, long-code analysis, or processing multi-page reports.
Llama 4 Maverick: The Workhorse for Advanced Reasoning
Key Highlights:
Enhanced Multimodal Capabilities:
Designed for general assistant and chat use cases, Maverick supports complex reasoning, creative writing, and coding tasks. Meta claims it outperforms models such as OpenAI’s GPT-4o and Google’s Gemini 2.0 on several benchmarks, especially in coding and multilingual tasks.Scalable MoE Design:
Maverick leverages a MoE framework with 128 experts. Despite having a total of 400 billion parameters, only 17 billion are active during inference. This strategy strikes a balance between scale and efficiency.Hardware Requirements:
While Maverick is powerful, it requires more robust hardware—running optimally on a single Nvidia H100 DGX system or equivalent.
Llama 4 Behemoth: The Internally Benchmarking Titan
Key Highlights:
Teacher Model for Distillation:
Though not yet released publicly, Behemoth is a massive model with 288 billion active parameters (and nearly two trillion total parameters). Its role is to serve as a teacher during the distillation process, guiding the performance improvements seen in Scout and Maverick.Superior STEM Performance:
Internal benchmarks indicate that Behemoth outperforms models like GPT-4.5 and Claude Sonnet 3.7 on math and STEM evaluations—paving the way for future AI research and development at Meta.
Mixture-of-Experts (MoE) Architecture
A defining feature of Llama 4 models is the shift to MoE architecture. Rather than engaging the entire model for every query, MoE allows only a specialized subset (“experts”) to process each token. This not only reduces computational overhead but also tailors processing power to the specific task at hand. For instance:
Scout’s Efficiency:
With 16 experts and a design that activates just 17 billion parameters per token, Scout can efficiently tackle tasks requiring a massive context—up to 10 million tokens—without unnecessary resource expenditure.Maverick’s Balance:
Maverick’s design with 128 experts ensures that even though the total parameter count is huge (400B), only the essential parameters are used for each query, keeping latency low while maintaining high-quality outputs.
Multimodal and Balanced Responses
Meta has also focused on enhancing multimodal understanding. All Llama 4 models are trained on vast amounts of unlabeled text, images, and video data, equipping them with a broad visual understanding—a capability that extends their utility beyond mere text-based tasks.
Moreover, Meta reports that the new models are tuned to respond to “contentious” questions more frequently and in a more balanced manner. This adjustment aims to address longstanding criticisms about political bias in AI models, ensuring that responses are both helpful and impartial across diverse viewpoints.
Licensing and Accessibility: Open but with Conditions
Despite being touted as “open source,” the Llama 4 models come with some noteworthy restrictions:
Geographical and Commercial Restrictions:
Users or companies domiciled in the EU are prohibited from using or distributing these models—likely a response to strict regional AI and data privacy laws. Additionally, companies with over 700 million monthly active users must obtain a special license from Meta.Developer Concerns:
These licensing conditions have sparked debate within the developer community, as some view them as potential barriers to the broader adoption and customization of the models.
Meta’s official blog post emphasizes that “these Llama 4 models mark the beginning of a new era for the Llama ecosystem,” even as it hints that this is just the start of what’s to come
Market Implications and the Competitive Landscape
Meta’s release of Llama 4 comes at a time when competition is heating up in the AI sector:
Responding to Chinese Competition:
The rapid development of open models from Chinese AI labs, like DeepSeek, reportedly spurred Meta into overdrive, prompting internal “war rooms” to understand and match the cost-efficiency and performance of these rivals.Positioning in the Open-Source Ecosystem:
While companies such as OpenAI and Google are leaning towards proprietary models accessible only via APIs, Meta’s decision to open-source its Llama 4 weights (albeit with restrictions) reinforces its commitment to fostering an ecosystem where startups, researchers, and even hobbyists can experiment and innovate without the hefty price tag.Integration Across Meta Platforms:
Already, Meta has integrated Llama 4 into its AI assistant features across WhatsApp, Messenger, and Instagram in 40 countries—with multimodal features currently limited to the U.S. in English. This broad deployment hints at a future where everyday applications are powered by cutting-edge AI, blurring the lines between research and real-world utility.
Conclusion
Meta’s launch of the Llama 4 series is a bold statement in today’s rapidly evolving AI landscape. By leveraging a mixture-of-experts architecture, the new models achieve a unique balance of scale, efficiency, and versatility. Whether it’s the lightweight and context-heavy Scout, the robust and versatile Maverick, or the internally benchmarked Behemoth, Llama 4 is poised to redefine open-source AI innovation.
However, the accompanying licensing restrictions and hardware demands also signal that while Meta is opening new doors for many, it remains cautious about the broader implications—particularly in regulated regions like the EU. As the AI community digests these developments, one thing is clear: Meta is committed to making high-performance AI accessible while carefully navigating the challenges of ethics, regulation, and competition.
Stay tuned as we continue to track updates on Llama 4’s capabilities, real-world performance, and its impact on the open-source AI movement.